AI in Venture: Who’s going to be left behind?
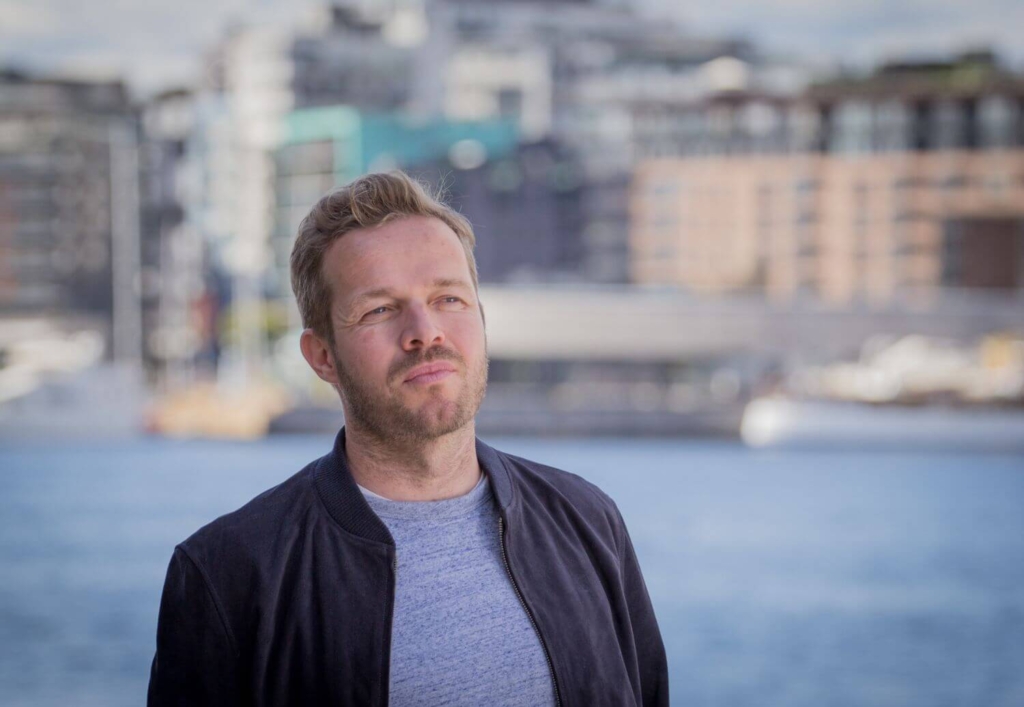
Two years ago, analysts at the research firm Gartner predicted that 75% of all venture investors will be using artificial intelligence in their investment decisions by 2025. That number already seems dated. If the prediction holds true, it means 25% are not doing their job.
Since the launch of ChatGPT, large language models (LLMs) have become available to everyone. Development has exploded, and Google CEO Sundar Pichai recently stated that no business can escape the trend. It’s hard to disagree. What does this mean for VC and venture investments?
Software has been sweeping the world for the past decade, largely driven by venture capital. Yet, it’s easy to be surprised at how little has happened in terms of developing the “tech stack” for colleagues involved in early-stage investments. As many have pointed out, the VC process innovation has been slow, moving beyond Excel spreadsheets has been slow. Until now.
AI isn’t coming to take away your job as an investor. Puh. But venture firms and investment teams armed with engineers and AI are. If the majority of the everyday job of investing becomes less valuable, the value of expertise increases.
What’s happening right now?
Two years ago, we began our first AI projects at Katapult. With grant support from both the EU and the Norwegian Research Council, and in collaboration with external AI experts such as Backen & Beck, we started building an internal data analytics team. The general experience is not surprising in that more or less all parts of the investment process can – and will – be improved, with speed picking up and new tools being launched by the day.
At Katapult, we can cast a wider net and search for companies in a multitude of global databases, “scout and screen” a larger number of startups, conduct deeper analyses more quickly, act with greater precision, and match funds and portfolio companies more quickly with strategically relevant investors – all with fewer resources and less time.
In this context, and in the wording of Andre Rettarah of Earlybird Venture, a burgeoning “class divide” is also emerging between “the old school” – investors who continue with “manual” investment processes as before, doing the job as they always have, “productivity VC” using off the shelf tech stack, and the new school of “Data-driven VCs” (DDVC) those who adopt and are ahead in the use and development of large databases, new language models, algorithm-based analysis tools, and above all, build up proprietary data and local expertise.
In the last six months, ChatGPT has accelerated development, new tools are being launched continuously, and the availability of advanced large language models (LLMs) allows even small venture teams to create sophisticated tools in the hunt for new global winners. And it is just the beginning.
Picking global winners
At its core, investing is about picking winners. For Katapult, this means picking global winners within climate technology. Fundamentally and in principle, this involves analyzing boundless amounts of unstructured and alternative information. It is in this landscape, new tools and services are launched to help find, analyze, DD, invest in and assist tech startups.
Only weeks ago, one of the largest VC databases, Pitchbook, launched its “VC Exit Predictor.” A tool that trains on both Pitchbook data and alternative data, giving startups a score for how likely it is that the company will be acquired, go public, or go under. Not an impressive tool in itself today (you can actually get more out of a GPT4 subscription and some basic prompting), but given the speed of development right now, we expect this and similar tools to quickly advance – and replace jobs.
Pitchbook uses data from active investors and investment activity, startups’ own performance indicators, proxy indicators of leadership and team competence, social media activity, business models, scalability in technology, growth figures, and metric models for engagement, retention, churn, and more. In addition to news articles, LinkedIn profiles and analyses of market position. In short, mostly the same metrics that a team of analysts manually apply. The difference is time, and what takes AI seconds, takes a team weeks and months of work, with greater sources of error, and slower learning.
“VC Exit predictor” is currently based on the companies being analyzed having raised two rounds of investments or more, and does not work on the earliest investments. Yet. Pitchbook already has one of the world’s largest databases, and a simple prediction is that network effects make the quality of it self-fulfilling. Startups will simply not want to have a low or incorrect score and will ensure updated data. The tool itself incentivizes updating your data, and the model is itself updated every 6 hours, fine-tuning increasingly advanced algorithms and becoming more precise by the day.
As a test, we entered Katapult’s portfolio companies, and even though the comparison is currently thin, the results look good. Not least, it’s pleasing to see Norwegian Portfolio Company, Chooose, topping the list with a solid “opportunity score” of 96.
The “Exit Score” is a simple example of what has emerged in recent months. With exponential growth in both development of, and access to, data, what will it look like in a year? Or 3? Or when your fund is due to be returned in 7-10 years?
What will be the effect of increasingly powerful and precise models? How does it affect investment decisions? Will they become self-fulfilling bubbles? Who will invest in companies with a low score?
To predict the future, you can look backward for similarities, or forwards for the acceleration of trends.
What can we learn from financial history?
First of all, we can learn that anything that can be quantified will be quantified and that qualitative and human evaluations and expertise will still be necessary. We can also learn that those who are early adopters will become the biggest winners. It’s in the nature of investing. More information, better analysis, and more data points provide advantages. Much of what is happening in venture development now, for example, we have seen before in hedge fund management. However, some aspects are new.
Since the 80s, and especially in the last 20 years, as more data became available and better analysis capacity developed, hedge funds have become increasingly dominated by quantitative modeling and investment decisions. Wall Street’s original “Gordon Gekko caricatures”, which relied on “instincts”, gut feelings, networks, and insider information, were quickly sidelined as data access increased. Insider information certainly still works, but the competence needed has changed.
As large amounts of public data became available and new databases were established, traditional fund managers increasingly hired algorithm and mathematical experts. Since the early 2000s, the growth in the share of algorithmic trading has increased from near zero, to an estimated over 75% of the total in 2023, and it continues to grow. Today, it is as obvious for hedge funds to base their analysis on “big data”, artificial intelligence, and algorithms, as it will be for venture tomorrow.
To be able to apply both new tools like LLMs, and not least the emergence of new databases – and pick better winners – we will see the introduction of “data scientist” teams in venture companies. In fact, it is already there, and the top 20 of the data-driven VCs already have an overall average of 10% engineers amongst all employees according to the Data-driven VC 2023 report.
There are two main reasons why venture is being disrupted right now. LLMs and a growing number of accessible databases.
Start-up and early-stage investments are characterized by both structured and unstructured data, with the latter becoming more prevalent the earlier the stage of the company. This unstructured data is often less accessible for analysis, which is why gut feelings, instincts, and experience are considered important investor traits. The cliché is true as having seen thousands of companies increases the chances of recognizing a well-founded team.
Systematically processing large amounts of data has traditionally been time-consuming, and for most fund models, it has been difficult to justify the time required, particularly for analysing a sufficient number of small companies in the early stages. The job has been too significant in relation to the company’s valuation.
Although there has been a significant shift of funds casting their lights on earlier stages, the rule so far has been that the larger the fund, the later the stage of investment. With more analysis tools available, and quicker and better analysis at hand, however, early-stage investing will likely increase across all funds.
About ten years ago, the first “big-data” wave hit the venture industry, and many predicted significant changes. Google Ventures, for example, was bullish about the future of startup investing in “big data.” However, little happened in the first few years, and it turned out that the data available was generally too limited. The joke being that those models were limited to measuring Twitter traffic as a signal of traction, or simple insights such as “successful founders breeding more success”. Initially, the models mostly delivered self-evident results.
The biggest change now is the emergence and quality of available databases like Pitchbook, Crunchbase, Dealroom, combined with the ability to easily set up one’s databases. Maybe most importantly, the many alternative data sources available, such as Peopledatalabs.com and Startup-insights.com. This provides easy access to analytical data on practically everything recorded about startups, even before they have a website.
Additionally, there is an endless supply of alternative data available, whether one wants to crawl LinkedIn profiles for experienced entrepreneurs with “Stealth” profiles, or Github or tech media, community groups, other incubators, accelerators, VCs, etc.
Picking winners in venture is both instinct and science and now, science is approaching the most experienced and instinctive gut feeling – just without biases and prejudices. The difference from just ten years ago is enormous, and with the emergence of LLMs and new databases, if 80% of the analysis can be done with new tools, the value of the remaining 20% becomes 100X.
Scouting, screening, and 100X deal-flow
For early-stage funds and investments, the majority of the most resource-intensive work lies in the sourcing and screening phase of finding companies.
At Katapult, we analyze an average of 100 companies per investment, and in 2022 alone, this involved identifying nearly 4,000 early-stage climate companies globally, which after the screening, interviews, and extensive due diligence, resulted in a total of 39 investments.
It is in this initial phase – scouting and early screening – that it is currently easiest to use large language models and apply gradually more automated analyses, and continuously to tune the algorithms to find relevant companies.
When a company is identified and enters our pipeline, we already have integrated tools that provide us access to everything from our proprietary “network score” that shows which investors and networks of investors are involved in the company, the number of employees and the composition of the founding team, the number and types of financing rounds that have been done, business areas and sectors, what kind of impact and sustainability goals they operate within, and simple summarizations of other available information about the company, etc.
And even more importantly, the pace of this development is fast, with new indicators being added weekly based upon experiences from previous rounds, analyses, and eventually also upon experiences in an increasing number of professional and discussion groups and articles that share playbooks for data-driven investment processes.
The time-consuming scouting process can be done faster and more accurately, with more accurate information available. Just by connecting our databases with GPT4 and a simple Google search – you can easily multiply the capacity of a “junior investment analyst”. The savings are easy to calculate, and even more importantly, the foundation for investment decisions are improved.
Which in the end is what increases both impact and alpha.
In-house or outsourcing?
Tools and solutions for accessing specialized deal flow are also the easiest to purchase externally. This allows even larger funds to move into the early stage, stop using expensive scouting consultants from the Big Five, and gain access to companies that match their investment strategy on a scale that was not possible only a year ago.
With tools such as Leadpicker, you can acquire easily accessible investment opportunities, relatively tailored to your investment strategy. However, buying the solution quickly creates a challenge in keeping track of the variables that you do not control. The “black box” of algorithms from external suppliers can help in the short term, but gives you limited added expertise, and you risk losing domain and competitive advantage. Training the algorithms on your own and partly proprietary data, in addition to external data, is necessary to differentiate your own funds.
To truly harness the advantages of AI and big data, it is necessary to match the investment team with AI and data analytics expertise. The everyday nitty-gritty of implementing new analytic tools alone requires more coding than is available in most investment teams. If 80% of the “manual” work can be automated, you are still dependent on building unique expertise that lies in the remaining 20%. As specialized impact investors, we, therefore, need to have control over the analyses “in-house” in order to use and leverage the new tools that are launched weekly. So the answer to the outsourcing vc in-house solution is a hybrid model.
At Katapult, we are not yet where scouting as a service company “Leadspicker” claims to be, where they replaced 35 junior analysts with automated processes and annually sort through nearly 100,000 startups for VC investors. Although with the pace of development, we see now, it may not be long until we get there.
Ultimately, and over time, the game is to deliver a better “alpha” than competitors. It simply does not work to follow the herd, fully rely on external expertise – or be left behind. Internal AI competence and environment must be built. At Katapult, we started this job a couple of years ago, have a solid AI team, are well underway in integrating the work into the investment processes, and experience first-hand how quickly development is progressing.
Investment strategy as “prompts”
With GPT4, the limitations in input (prompt) and output (response) have gone from 3000 to 25,000 words in a few months. You can now feed in most of your investment strategy, the criteria you use, and match it against easily available data. Alternatively, you can have it read through the entire pitch deck.
Language models like GPT4 and Google’s Bard are generalists and can easily pass most university exams, speak most languages, and have access to most of what is written online. To manually compete, you need to have seen a few thousand startups, and look away from all of your biases.
With reasonably well-structured data, you can go further and fine-tune GPT models against your own databases, and you can do the same for individual companies, or build insights from the “anti-portfolio,” and systematize experiences from those you didn’t invest in.
If you do DDs, you can similarly do initial quality checks against common business models, quality check TAM models, or you can, for example, take Andreessen Horowitz’s (a16z) playbook for growth metrics and analyze growth based on well tested models. Or you can quickly get an overview of the competitive landscape, characteristics of other similar companies and/or the investors financing them. In short, with simple tools, you can already get answers in seconds – that only last year took weeks.
“Motherbrain”
EQT was a relatively early adopter in this game and has achieved a lot of attention with its smartly named “Motherbrain” project. It was launched as early as 2016 and has been developed into an internal general support for investment decisions. One of Motherbrain’s key services is to support the acquisition of smaller companies as strategic add-ons to existing ownerships, and in analyzing complementarity in relation to products and services.
As for early-stage investments, they have used language models (LLMs) to scan thousands of companies to more accurately and quickly find good matches for M&As. The process simply consists of the investment team describing the characteristics and criteria for the companies they are looking for, then getting the proposals, and swiping right or left for further and more manual analysis.
The experiences from EQT’s development are aligned to those of Katapult. For the analyses to be useful, investment in a team that actually knows the technology is required so that the analyses are transparent, the results are traceable, and the insights from the investment expertise in the investment team can be fine-tuned. Black box processes and blind use of technology processes are poorly suited for investment analysis. You need customization, and currently, it can be compared to cycling up a steep hill with or without an e-bike.
Therefore the data engineer vs. investment analysts ratio is a good indicator for LPs, and at Katapult it is quite far up on the top list globally with 3/15.
Global investor networks – warm introductions on steroids
Venture capital is largely driven by network logic. Warm introductions, large networks, and personal relationships have always been crucial. Warm introductions provide quick trust in verifying whether the information is correct and a shortcut to quality-assured information. It is also the main reason why local ecosystems are important, and why Silicon Valley, as the global example, has gained a mythological position. Tight investor networks that know each other provide shortcuts to smart capital, which in turn gives companies rocket growth.
The numerical challenges are obvious. 99,9% of investors are not in your network. It is simply more likely than not, that the best investor fit for your fund or portfolio company is somewhere else. With an almost exponential growth in VCs globally, the challenge increases by the year.
The limitations of personal networks simply relates to the size of the world, and the rapid growth of the number and spread of well-functioning ecosystems. New winners are coming to an ever-greater extent from new geographic areas. Counting unicorns alone, in 2022 they were bred in more than 100 ecosystems globally. In Katapult this is reflected in our portfolio where we have a total of 146 companies from 47 countries.
In our recently developed databases, we now have over 200,000 investors from all over the world, and we can connect portfolio companies or our own funds with contacts and information from any of these in seconds. Using large language models and these databases, we can find the 2, the 10, the 50, or the 100 most active and competent investors in a specific field, what stage they invest in, who they co-invest with, common ticket size, key points of the investment strategy, knowledge and contribution, and most other data that a few years ago was only available if you had close and personal contact.
The point is not that AI will replace warm introductions, but the logic of warm introduction, easy access to trustworthy information, is being challenged. With new tools it is possible to build investor networks on steroids. You can spend less time on random conferences, meetups and calls, proportionally more time on building and nurturing relationships with those who have the best strategic match. And not least, be way quicker in finding emerging and existing investors that match your strategy.
In this way, AI already gives us a super muscle in what is the most important job in addition to finding the winner: finding strategically correct investors who ensure further growth, and not least investors and LPs who match our investment strategies and funds.
There are thousands of them globally, only a fraction are found in your own local ecosystem or existing network, and chances are the best match is not where your bias used to take you.
Language models and new databases function as spotlights into a global network of investors that always surpass the randomness of personal networks. They are not replacements, but they can be built faster and much more accurately. Warm introductions become even warmer when they are precise and data rich. If you get a precise recommendation from someone who has done their homework – preferably with the help of ChatGPT – the relationship also stays warmer than when you get yet another introduction from someone who always gives tips that are a bit halfway.
Just 10 years ago, the majority of successful tech startups came from Silicon Valley. It was also where the leading VC communities were. Since then, solid ecosystems have emerged all over the world, and in the EU alone, almost 100 new VC funds were established in 2022 alone. 10 years ago, it was also possible to build a globally leading network by attending after-work events on Sand Hill Road once a week. Although Sand Hill Road is still more important than Wall Street for financing tech startups, that time is definitely over.
The Tech Hype – Is this time different?
It’s difficult for the human brain to grasp “the power law” and understand the exponential patterns that characterize most new technologies. And even though it’s theoretically understood and modeled, history shows that realization comes too late. In the many tech revolutions that have come in the last twenty years, people have become “tired” and numb to “revolutionary” technology, and a lot of new things are launched with the same “hype” rhetoric every year.
VR and augmented reality were supposed to give us the Metaverse and new visual interfaces, Web3, Blockchain, and crypto were supposed to democratize creative industries and ownership of digital production, and we can add an endless series of new “tech phenomena” that have become different to their hype. Although AI has been high on the list for a couple of decades, right now GPT4, Bard and DallE are leading the way, made use available for “all”, and realization took off. So what is the hype and what is reality?
When messing with language, the most fundamental tool for human meaning, knowledge and existence, some technologies simply represent more fundamental changes.
When AI directly affects language, and does the job better than most people in more and more areas, it’s something else than just innovative means of production, a new productivity tool, or yet another industrial revolution.
It’s simply more fundamental.
Who will be left behind?
According to expert researchers and some of the best AI developers, everyone will be left out. Some of the leading research and development communities in the world recently called for the further development of the most powerful models to be halted until better regulation is in place. It is easy to predict that it won’t happen. Still, the prevailing view among experts is that we are approaching ‘AI as an existential threat’.
Simplified, the reasoning is: when we create artificial intelligence that is able to make itself smarter, we lose control. Then it’s no longer the programmer who is programming, but the models themselves. If we measure the development of data power in “FLOPS” (Floating-point Operations Per Second), “old Moore’s Law,” which predicted that data power doubling would happen every 20 months, this reasoning has now been surpassed. We are now down to every 6 months. At the same time, the availability and production of data are exploding, and that is the core. Faster computations, combined with more data, creates faster computations and more data. The question then arises as to whether we will still have control?
Available databases for information on startups and investors, the quality of these, and ease of use, have exploded in the last year. We also know this development will not slow down, and one doesn’t have to believe the most dystopian researchers and experts to understand that also the core of venture and VC investments will change forever.
Analyzing companies and the knowledge needed to pick winners, matching portfolio companies or your own funds with the right investors, and most importantly, developing individual companies and monitoring portfolios are all areas that are changing extremely rapidly. Measured in with the timeline and maturity of a VC fund, no one is even close to seeing the implications.
Algorithms and large language models eat business models for breakfast, and jobs for lunch, and create at least as many new ones. The only thing that’s certain is that if you don’t jump on the train and invest in the tools, you will be left behind at the station. Then you’re out.
Fredrik Winther is Katapult’s Chief Strategy Officer and Partner. Stay up to date on the latest Katapult news, here.